Emotions: The New Variable In Shaping Effective Algorithms
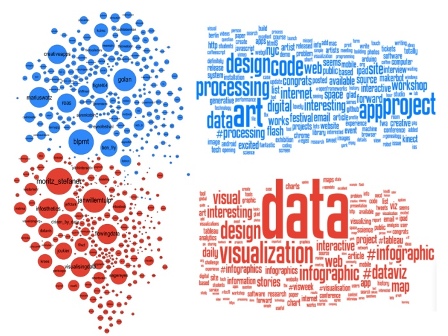
We thought of everything when it comes to analyzing big data and understanding how to master the art of efficiency both online and off. But one important thing is hard to plug into the equation: the mood of employees and the customers we serve. The mood of a given demographic or unique account has to be considered, and figuring out how to predict that means analysts who aggregate and try to make sense of raw data to generate algorithms have to be a lot more perceptive than we previously could have imagined.
One of the best examples of this is based on human interactions between sales departments and the clients they interact with. Think about the show “The Office”, for example. The sales staff at Dunder Mifflin paper company are often caught in funny scenes while on the phone with clients—the mood of those clients determines how long sales staff is on the phone, how much “shooting the bull” is part of their interaction with the client, and how much of a simpatico relationship they have with the client at hand. Conversely, some clients served may be all business and may not be at all interested in small talk, making their conversations especially curt. So how do we take the personalities and day-to-day feelings of this emotionally diverse business culture into account when deciding on a quota, daily or monthly goals, and algorithms for reaching these clients by phone call, email reminder, or mailers?
The software we use to predict how long interactions will last, and how loyal customers are (or aren’t) means we have to consider their sincerity as well. The prime example of this is ecommerce. How often do people visit Amazon.com, find an item or two they want, and then wind up leaving the site to check on prices elsewhere, leaving their Amazon shopping cart full? Smaller online entities face the same hardship, and this makes it difficult to decipher what we can be doing better to keep them on our websites, fill those shopping carts, and ultimately buy whatever it is they’re looking for from us rather than continuing to surf the web looking for better deals. The place we have to start is analyzing how many people are placing items in their shopping carts, what kinds of items they are, and how quickly they bounce from the site. Big data helps us see these interactions, and can help us decide on various tactics to keep this unfortunate situation from happening as often.
One of the most effective ways to curb sales loss is to take a page from Amazon’s book—rather than simply deleting items when a person logs back into their account, Amazon keeps these items in the shopping cart with the option to add on to a new order. In addition, customers visiting Amazon also have the option to add what they want to their “wish list”—in this way, people are reminded of what they wanted the last time they were on the site, and can choose to buy or not buy. But this paradigm works in another great way: Amazon (and those who opt to implement a similar strategy) don’t have to be confused by shopping carts left to twist in the wind, making the analytics of big data regarding customer behavior and mood easier to understand and manage.
When it comes to managing employees, we meet a similar conundrum. For example, just because we create a work schedule a week ahead doesn’t mean all employees see it—maybe their next scheduled shift occurs three days after said schedule was made, and allowances have to be made for efficient shift coverage. This may not be a huge deal for a mom and pop coffee shop, but for a massive corporation like Walmart and operations that employ dozens or even hundreds of employees, a scheduling conflict can upset the apple cart in a pretty serious way. Employee mood, playing hooky, and unforeseen emergencies in the lives of these employees have to be considered. To this end, analyzing time sheets for big operations means in-depth digging through big and small data to discover the best ways to create schedules that will stand up to the variables caused by the human condition.
Related Reading:
Business Intelligence and Analytics for Big Data | QlikView
A Beginner’s Guide to Big Data Visualization | SmartData Collective
Choosing the Right Business Partners Using the Gartner Magic Quadrant – Yahoo Small Business Advisor