BIG DATA ANALYTICS‘BIG’ TRENDS FOR 2017
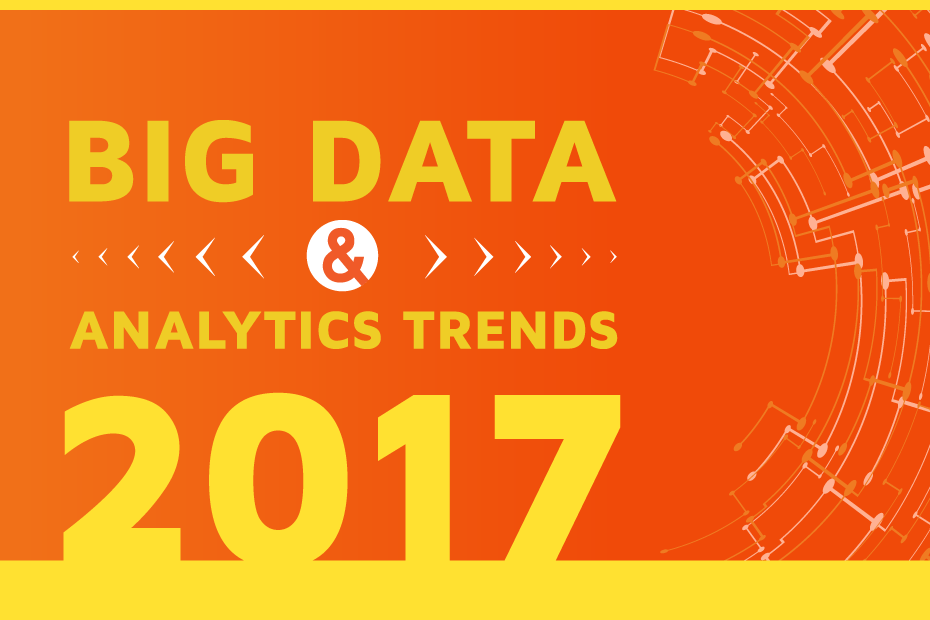
With the advent of big data analytics came myriad advancements in technology and data science including cognitive computing, cloud data services, and predictive business models. The applications of big data analytics and data science have been reshaping business processes and companies. In 2016, companies moved projects of data management and analytics into production in order to interrogate both external and internal data to know their customers and improve efficiency.
Though the most surprising trend of 2016 that no expert saw coming was a sudden decline in Map Reduce, one of the core components of data management and processing engine Hadoop- which is still a mainstay for data cleaning, acquisition, and cleansing of unstructured data, 2017 will definitely have a lot more surprises in the store. Here are some picks for the ‘hottest’ big data analytics trends in 2017-
Big data era is here and it has a lot to offer than just analytics and data management for corporate space. The entire planet and even the interstellar space will be monitored, sensed, optimized and managed by cognitive IoT- Big data analytics is definitely going to present even ‘bigger’ opportunities in the coming years! All hail data science!